DeepSeek Surpasses ChatGPT as Most Downloaded AI App
- CoinLink
- Jan 31
- 3 min read
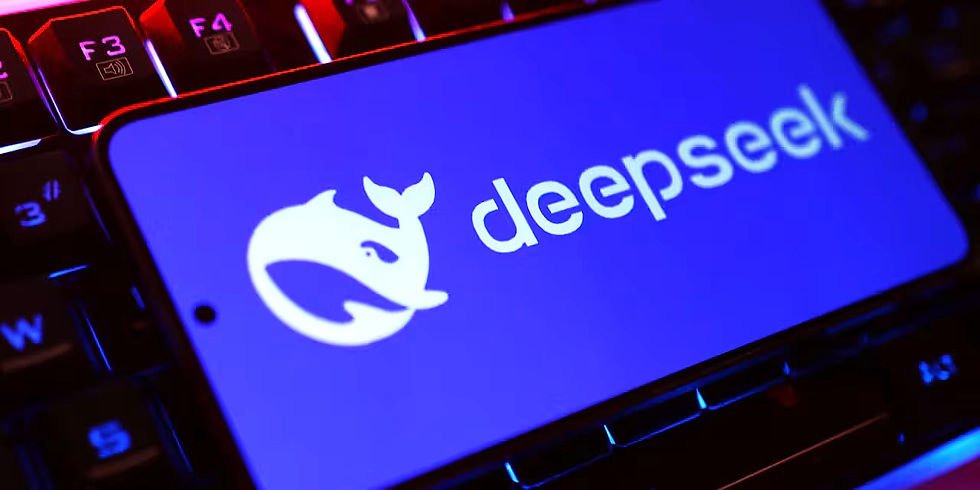
Chinese AI firm DeepSeek has gained significant attention after surpassing OpenAI’s ChatGPT as the most downloaded app on the Apple App Store. This achievement follows the release of research papers in which DeepSeek demonstrated that its latest R1 models, developed at a significantly lower cost, match or even surpass OpenAI’s strongest publicly available models.
How DeepSeek Achieved a Competitive Edge Over OpenAI
Determining the exact reasons behind DeepSeek’s success is challenging since OpenAI has not disclosed much about its GPT-4o and o1 training processes, which previously set benchmarks across multiple AI tests. However, clear distinctions exist between the companies’ approaches, and DeepSeek appears to have introduced several important innovations.
The most significant difference lies in efficiency. DeepSeek has developed high-performing models with substantially lower computational costs, a factor that caused a drop in the stock prices of chip manufacturers like NVIDIA at the start of the week.
DeepSeek’s latest R1 and R1-Zero models are built upon its V3 base model, which the company states was trained for under £4.7 million using older NVIDIA hardware. These components remain legally available to Chinese firms despite U.S. restrictions on cutting-edge semiconductors. In contrast, OpenAI’s CEO, Sam Altman, has stated that training GPT-4 cost more than £78.6 million.
Karl Freund, founder of Cambrian AI Research, told Gizmodo that U.S. policies limiting access to advanced chips have forced Chinese companies like DeepSeek to optimise their AI models rather than invest in costly hardware and large-scale data centres.
“You can build a model quickly, or you can put in the effort to build it efficiently,” Freund said. “Western companies will now have to do the difficult work they have previously avoided.”
DeepSeek’s Approach to Optimisation and Training Efficiency
DeepSeek has not invented most of the optimisation techniques it employs. Several strategies, such as using memory-efficient data formats, have been explored by larger competitors. However, DeepSeek’s research suggests a team dedicated to incorporating every available method to reduce computational memory usage while ensuring maximum efficiency on older hardware.
OpenAI first introduced reasoning models, which use a method called chain-of-thought to simulate human trial-and-error reasoning. This approach has proven particularly effective in solving complex mathematical and coding problems. OpenAI, however, has not disclosed the specifics of how this process was implemented.
DeepSeek has taken a different approach by openly documenting its methodology.
Generative AI models have traditionally improved through reinforcement learning with human feedback (RLHF). In this process, human reviewers assess AI-generated responses and identify desirable qualities such as accuracy and coherence. The model is then trained to favour these characteristics.
DeepSeek’s major breakthrough was eliminating human feedback and designing an algorithm capable of recognising and correcting its own mistakes. The research team stated that DeepSeekR1-Zero demonstrates self-verification, reflection, and extended reasoning abilities, representing a significant step forward in AI research. They further highlighted that this model is the first openly documented example of a large language model developing reasoning capabilities purely through reinforcement learning.
Challenges and Refinements in Training
Despite these advances, the pure reinforcement learning approach had limitations. The R1-Zero model’s responses occasionally lacked clarity and unpredictably switched between languages. To address this, DeepSeek introduced a refined training pipeline that incorporated a small amount of labelled data alongside multiple reinforcement learning stages. This hybrid training method produced the R1 model, which outperformed OpenAI’s GPT-4o in human-level mathematics and coding evaluations.
China’s AI Strategy and the Impact on Western Companies
Bill Hannas and Huey-Meei Chang, experts in Chinese technology and policy at the Georgetown Center for Security and Emerging Technology, noted that China closely tracks Western technological advancements. This strategy has helped Chinese firms develop alternative solutions to U.S. policies designed to maintain American dominance in AI research.
DeepSeek’s success represents an industry shift rather than a disadvantage for Western AI companies. Hannas and Chang suggest it highlights the need for AI firms to reconsider large-scale, high-cost solutions. The effectiveness of DeepSeek’s efficiency-driven approach demonstrates that innovation can come through optimised design rather than excessive computational power, an ethos evident in several state-backed Chinese research laboratories.
Comments